StyleDrop: Google's Game-Changer Ignites the AI Art World
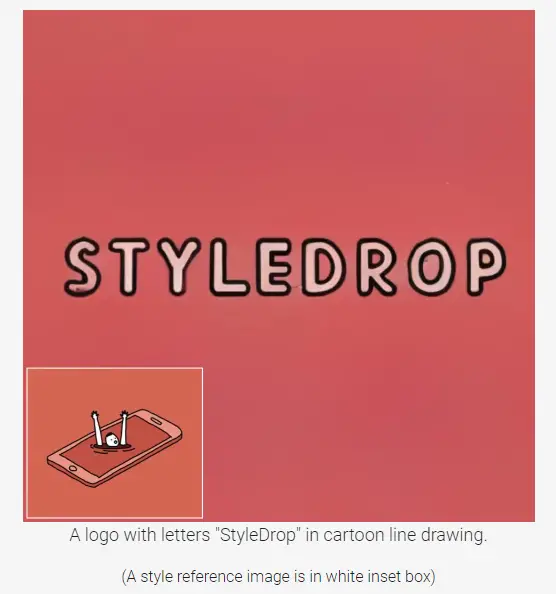
What is ChatGPT?
StyleDrop is a newly launched project by Google, utilizing two artificial intelligence models, Muse and CLIP, to implement a new text-to-image technology. This technology can generate images of a specific style with high fidelity. StyleDrop has a wide range of applications and can generate images based on subtle style differences and details provided by the user, such as color schemes, shadows, patterns, local characteristics, and globally applicable effects. The principle of StyleDrop is to learn new styles efficiently by adjusting a minimal number of parameters, then improve the quality through iterative training with manual or automatic feedback. Impressively, even if the user only provides a single image in a specific style, StyleDrop can still generate stunning results.
Price: Free
Tag: AI Art Generator
Developers:Google
Share
Inspiration source
According to the paper’s author, StyleDrop draws inspiration from a tool called Eyedropper, which is used for selecting colors from images. Just as Eyedropper allows for easy color selection, StyleDrop aims to provide a similarly convenient way for people to choose desired styles and generate corresponding patterns.
With StyleDrop, users can quickly capture and extract the visually appealing style elements they are interested in from one or several reference images, whether it’s unique textures, color combinations, or shape features. By transforming reference images into actual patterns, StyleDrop offers users a powerful tool in the creative and design process, encouraging them to explore new styles, create unique visual expressions, and translate these inspirations into real-world artworks.
Features of StyleDrop
- StyleDrop, utilizing the Muse text-to-image model, can accurately replicate and generate images of a specific style from a single input image.
- Through fine-tuning a minimal number of parameters and iterative feedback training, StyleDrop’s learning process is both fast and efficient.
- StyleDrop is capable of capturing and controlling subtle differences in texture, shadows, and structure, greatly enhancing style control capabilities.
- StyleDrop can be combined with other tools, enabling designers and companies to use their own brand assets to rapidly produce prototypes in unique styles.
How does it work?
The way StyleDrop works is by efficiently learning a small amount of trainable parameters (less than 1% of the total model parameters), and improving its quality through iterative training with human or automatic feedback. Interestingly, even if a user only provides a single image of the desired style, StyleDrop can still produce impressive results. Extensive experiments have shown that StyleDrop outperforms other methods in adjusting the style of text-to-image models on Muse, including DreamBooth and TextualInversion on Imagen or Stable Diffusion.
The figure shows some sample images generated by the StyleDrop algorithm. The image on the far left is a sample, representing the style the user wishes to transform. The resulting images on the right are generated based on the text prompt information, with a style similar to the sample image. By simply modifying the target in the text prompt, users can quickly obtain new images.
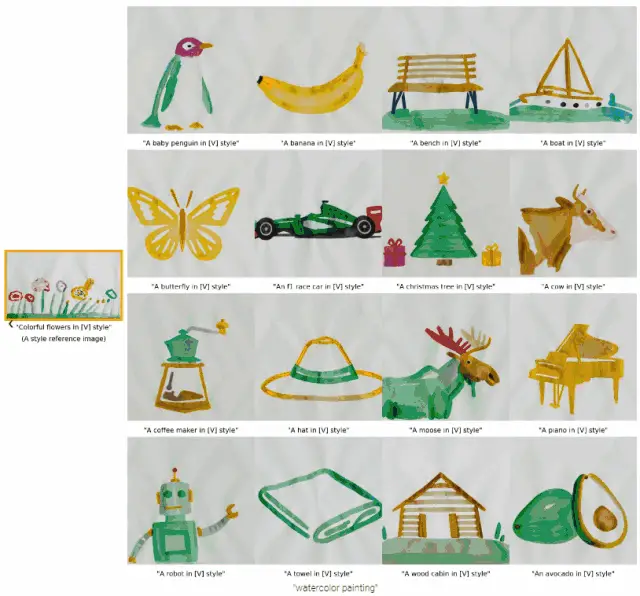
StyleDrop algorithm process
- Description Input: The user provides a text prompt, such as “a black cat sitting on a red sofa”.
- Choose or Input Style: The user chooses or inputs a style (like “Van Gogh’s Starry Night”) to be applied to the image to be generated.
- Style Encoding: The selected style is encoded into a vector using a well-trained style encoder.
- Text Encoding: The input text is encoded into another vector using a trained text encoder.
- Encoding Fusion: The style vector and text vector are combined into a single vector.
- Decoder Inference: The combined encoded result is converted into an image using a well-trained decoder.
- Image Output: The generated image, incorporating the selected style and the content of the input text description, is outputted.
Details about the StyleDrop algorithm
- Data Collection – The authors have gathered a diverse set of images in styles such as watercolors, oil paintings, flat illustrations, 3D renderings, and sculptures of varying materials, extending the visual style diversity beyond the main focus of neural network style transfer research.
- Model Configuration – The StyleDrop algorithm was built upon the Muse model and the method of adapter fine-tuning. In all experiments, the adapter weights were updated for 1000 steps using the Adam optimizer with a learning rate of 0.00003. The second-round model, referred to by the authors as “StyleDrop,” was trained using up to 10 synthesized images and human feedback.
- Adapter Configuration – An adapter was applied at every layer of the Transformer. Specifically, two adapters were applied after every layer’s cross-attention module and MLP block. The authors provided sample code illustrating how to apply an adapter to the output of the attention layer and generate adapter weights. All uplink weights were initialized to zero, while downlink weights were initialized from a truncated normal distribution with a standard deviation of 0.02.
- Shared Weights – The authors generated adapter weights in a parameter-efficient manner by sharing weights between the layers of the Transformer. This can be triggered by setting is_shared to True and can drastically reduce the total parameter count of the adapter weights. While these settings were used in all experiments by the authors, it is possible to easily reduce the parameter count without compromising model quality by setting is_shared to True.
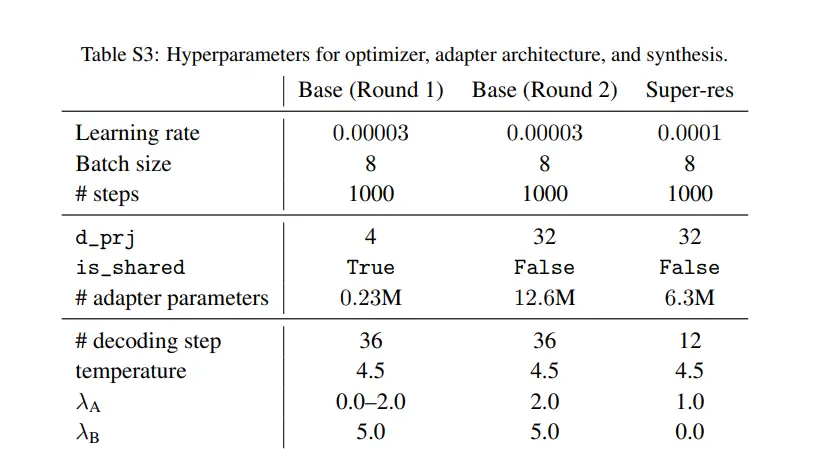
Performance comparison of StyleDrop algorithm
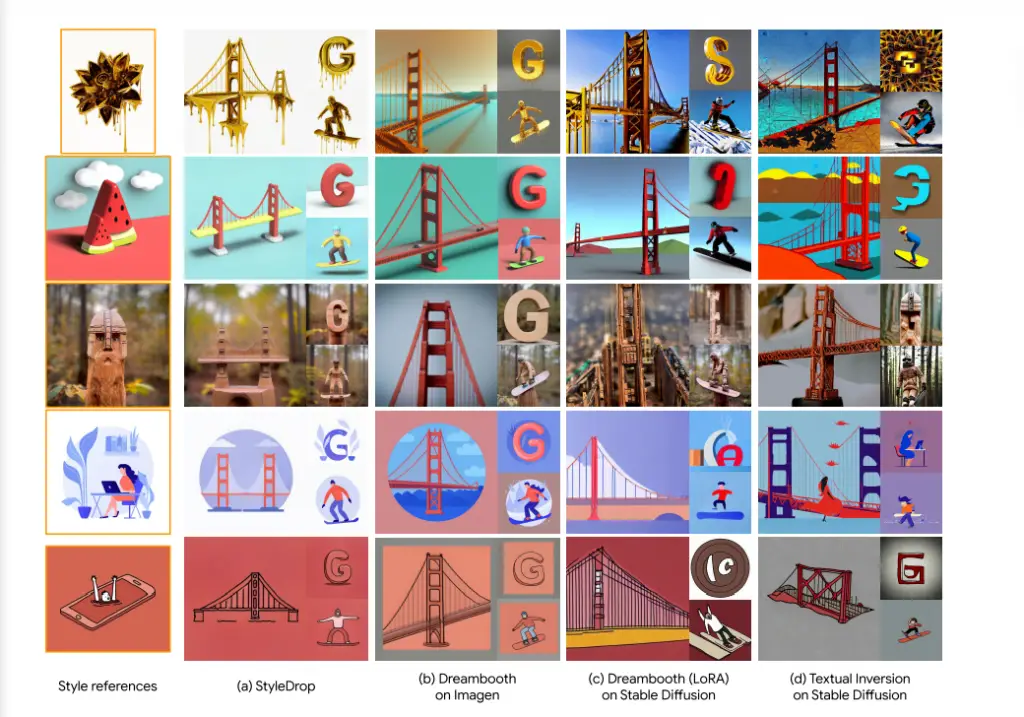
The figure above illustrates the generated results of the StyleDrop algorithm compared to other algorithms. The first column shows the original style reference images. The second column displays the results generated by the StyleDrop algorithm using the same text prompt. The third column shows the results generated by the Dreambooth algorithm with the same text prompt. The fourth column represents the results generated by the LoRA algorithm with the same text prompt. The fifth column represents the results generated by the Textual Inversion algorithm with the same text prompt.
Through careful observation and analysis, we can draw the following preliminary conclusions:
- Compared to other algorithms, both StyleDrop and Dreambooth algorithms produce more realistic and lifelike images.
- Upon closer examination of certain details, the StyleDrop algorithm appears to outperform the Dreambooth algorithm. For instance, in the first row, there is a higher consistency in the background color of the generated image using StyleDrop. In the second row, the details such as the “G” and the white cloud base of the bridge exhibit better quality in the StyleDrop-generated image.
These initial findings suggest that the StyleDrop algorithm exhibits superior performance in generating images with realistic and high-quality details when compared to the Dreambooth algorithm.
Examples of product
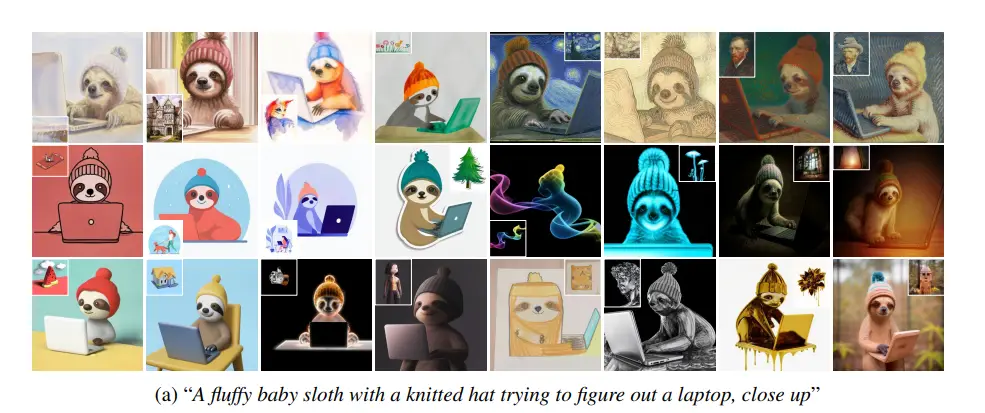
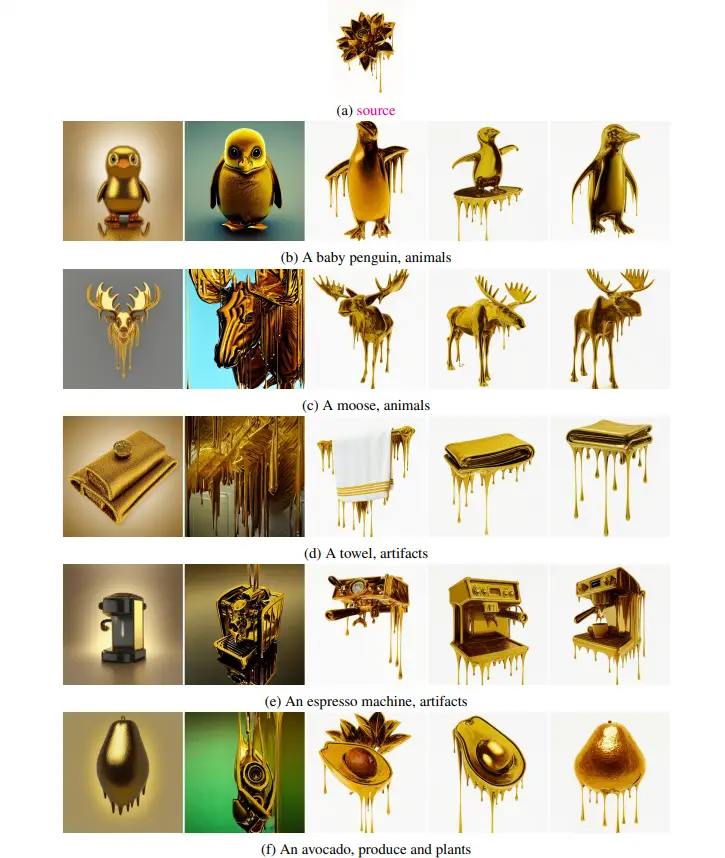
Conclusion
It is clear that Google’s StyleDrop is a significant innovation in the field of AI art. It has the potential to herald a new era of artistic creation and to profoundly influence the way we understand art and innovation. In the world of relentless competition and innovation, other companies, including Midjourney, will need to face this formidable contender.
FAQ
StyleDrop is a newly launched project by Google
Google is not yet available to the public, no pricing information for StyleDrop yet.