Textual Inversion in Stable Diffusion Step-by-Step Guide
In the realm of artificial intelligence, the ability to generate images from text prompts has opened up a new frontier of creativity. However, the potential of these models is often limited by the user’s ability to describe unique or novel concepts. This is where the concept of Textual Inversion in Stable Diffusion comes into play. It provides a simple yet powerful approach to overcome these limitations and unlock the full potential of text-to-image models.
Table of Contents
What is Stable Diffusion?
Stable Diffusion is a type of generative model that has shown remarkable capabilities in synthesizing novel scenes using text prompts. The model works by turning any input prompt into embeddings, which are then fed to the diffusion model as guidance or conditioning. This process involves tokenization of input prompts into a set of tokens, which are then passed through a text encoder to get embeddings. Each token is linked to a unique embedding vector that can be retrieved through an index-based lookup.
See more:10 Best AI Image Generator 2023
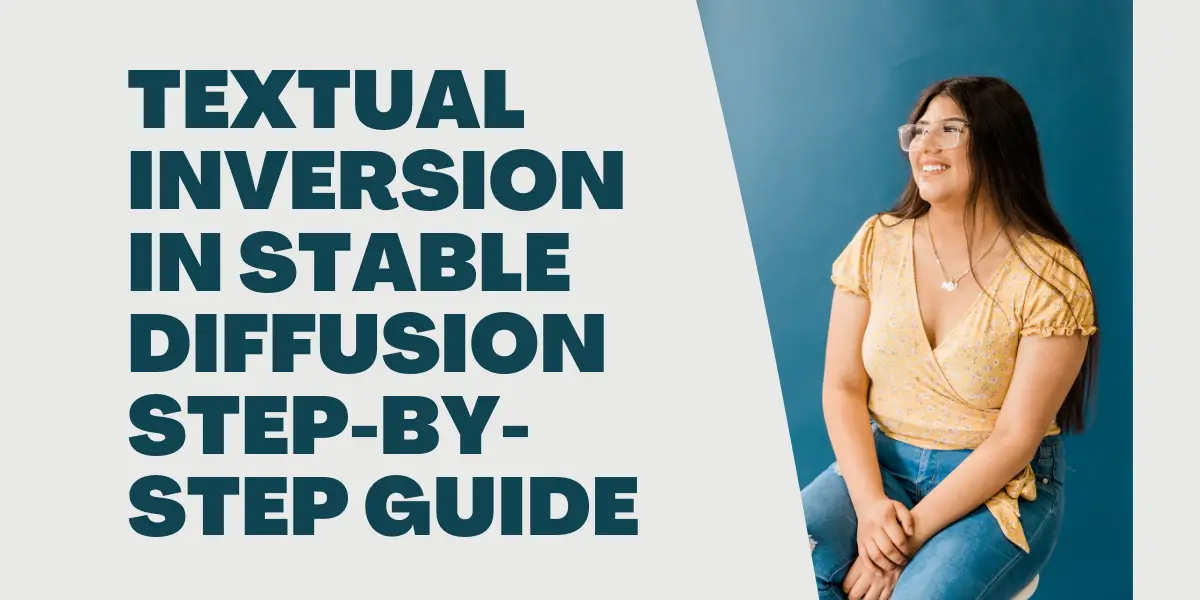
What is Textual Inversion in Stable Diffusion?
Textual Inversion is a method that allows users to teach new concepts to the text model using a few example images. The idea behind Textual Inversion is to use these images to train the embeddings of a new token, which is added to the vocabulary. This new token, or pseudo-word, represents the new concept that the user wants to introduce. Once trained, this pseudo-word can be used like any other word to compose novel textual queries or new sentences for the generative models.
How to Set up Textual Inversion in Stable Diffusion
Setting up Textual Inversion in Stable Diffusion involves a few steps:
- Choose a placeholder string, denoted by S*, to represent the new concept you wish to learn.
- Replace the vector associated with the tokenized string with a new learned embedding, v*.
- Use the pseudo-word like any other word to compose novel textual queries or new sentences for the generative models.
How to Get Textual Inversion to Stable Diffusion
Step 1: Collect a small set of images (typically 3-5) that depict your target concept across multiple settings. The quality of these images is more important than the quantity. Providing enough details for AI to understand the subject is crucial in training and generating accurate images.
Step 2: Choose a placeholder string, denoted by S*, to represent the new concept you wish to learn. This placeholder string will be used to create a new token in the vocabulary.
Step 3: Replace the vector associated with the tokenized string with a new learned embedding, v*. This new learned embedding is found through direct optimization by minimizing the Latent Diffusion Model (LDM) loss. Choosing the appropriate learning rate is crucial in this step, as it determines the flexibility of the model to apply any style while still being close to the subject being trained.
Step 4: Randomly sample neutral context texts like “A photo of S*”, “A rendition of S*”, etc to condition the generation. These context texts will be used to train the new token.
Step 5: Train the model with the new token and the set of images. The training process involves encoding these novel concepts into an intermediate representation of a pre-trained text-to-image model. The embeddings for the new token are found through an optimization process, which is referred to as “Textual Inversion”. This process is realized by re-using the same training scheme as used by the original Latent Diffusion Model, while keeping both c(θ) and ε(θ) fixed.
Step 6: After the training process, use the pseudo-word like any other word to compose novel textual queries or new sentences for the generative models. The AI in stable diffusion uses prompts to train images, allowing users to customize the training process and experiment with their own prompts.
Step 7: Evaluate the performance of the new token. AI-generated characters can be optimized by determining the step at which they start to look bad, potentially saving time and resources in the training process.
Step 8: Once satisfied with the performance of the new token, you can now use it to generate novel scenes that accurately depict the new concept you introduced.
How does textual inversion work?
Textual Inversion works by adding a new token to the vocabulary and training it with a few representative images. This new token, or pseudo-word, is then used to represent the new concept. The embeddings for this new token are found through an optimization process, which is referred to as “Textual Inversion”. The goal of this process is to find a single word embedding that will lead to the reconstruction of images from the small set when sentences of the form “A photo of S*” are used.
How to Training?
The purpose of Textual Inversion is to enable prompt guided generation of new, user-specified concepts. The training process involves encoding these novel concepts into an intermediate representation of a pre-trained text-to-image model. The embeddings for the new token are found through an optimization process, which is referred to as “Textual Inversion”. This process is realized by re-using the same training scheme as used by the original Latent Diffusion Model, while keeping both c(θ) and ε(θ) fixed.
Also read:What is Stable Diffusion Stock and How to Invest it?
Conclusion
Textual Inversion in Stable Diffusion is a powerful tool that allows users to introduce new concepts to text-to-image models. By using a few example images, users can train the embeddings of a new token, which can then be used to generate novel scenes. This method not only expands the capabilities of text-to-image models, but also allows for a more personalized and creative use of these models.