Details About Apple’s Secret Open Source Multimodal LLM ‘Ferret’
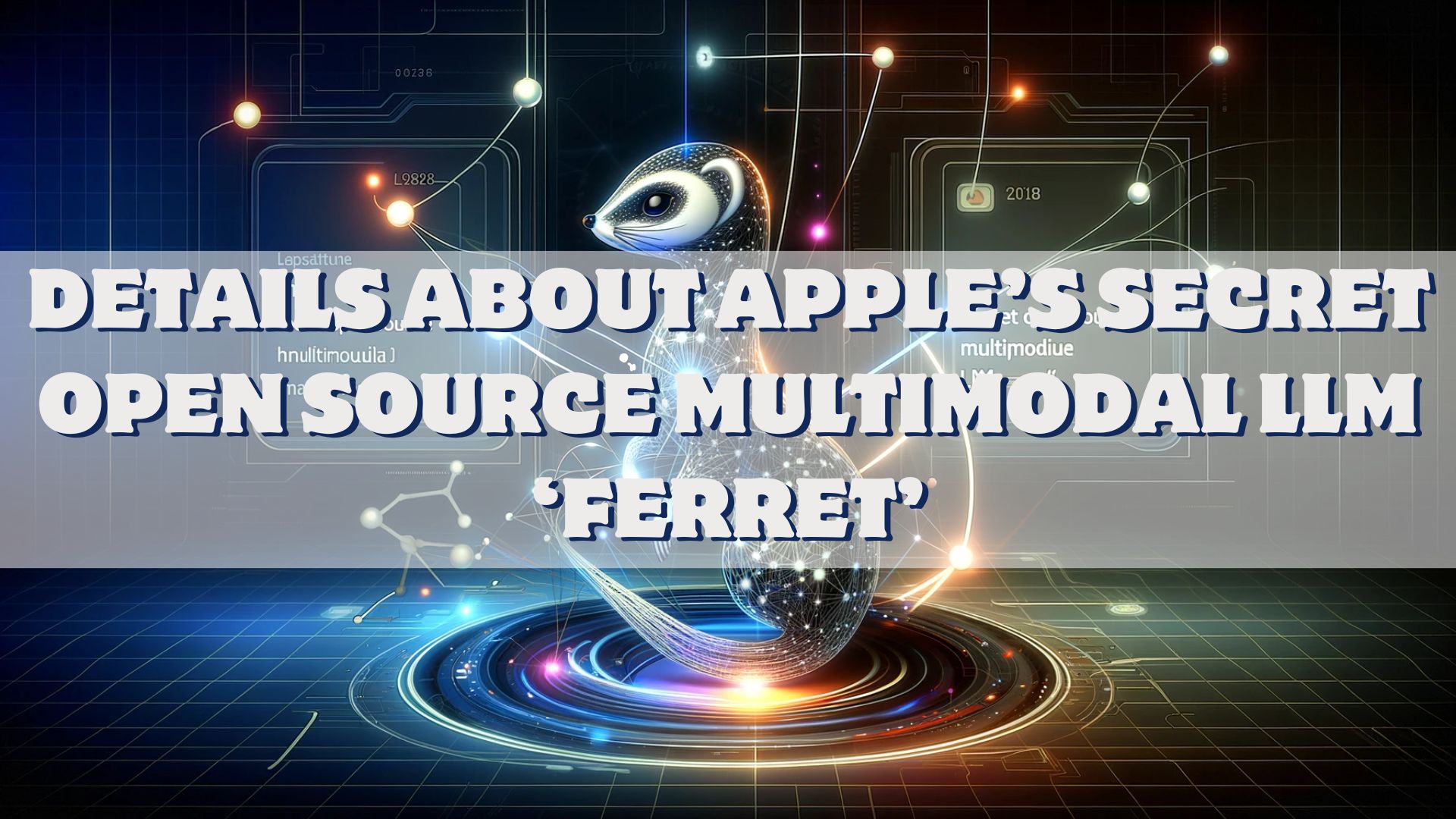
In the ever-evolving world of AI, Apple has made a quietly spectacular move. Just a couple of months ago, in collaboration with Columbia University, Apple unveiled ‘Ferret’ – a new multimodal Large Language Model (LLM) that’s open-source, a rarity for a company known for its closely guarded secrets. This move, almost like a whisper in the tech community, marks a significant shift in Apple’s approach to AI development and its potential impact on the industry.
Ferret is not just another LLM; it’s a testament to Apple’s commitment to impactful AI research, solidifying its place in the multimodal AI space. What makes Ferret stand out is its ability to refer to image regions in any free-form shape and automatically establish grounding for text deemed groundable by the model. This capability pushes the boundaries of AI, opening up new possibilities in image search, accessibility, and nuanced contextual understanding.
The introduction of Ferret, especially in an open-source format, is a strategic move that speaks volumes about Apple’s dedication to staying at the forefront of AI technology. It’s a blend of innovation, strategy, and perhaps a hint of Apple’s new direction in the AI domain. This article dives into the details of Ferret, exploring its capabilities, potential applications, and the implications of Apple’s foray into open-source AI.
Table of Contents
What Is LLM And What Can It Do?
Large Language Models (LLMs) like Ferret represent the cutting edge of artificial intelligence. These models are designed to understand, generate, and interact with human language in a way that was once thought to be the exclusive domain of human intelligence. LLMs can process vast amounts of text, learn from it, and then apply this learning to perform a wide range of language-related tasks.
The capabilities of LLMs extend from simple tasks like translation and summarization to more complex ones like writing articles, composing poetry, or even generating computer code. They can engage in conversations, answer questions, and provide insights based on the data they have been trained on. The versatility of LLMs makes them valuable in various fields, including customer service, content creation, education, and research.
What Is Apple Ferret LLM?
Apple’s Ferret LLM is a groundbreaking addition to the world of LLMs. Developed in collaboration with Columbia University, Ferret is a multimodal LLM, which means it can understand and interpret not just text but also images. This dual capability allows Ferret to analyze specific regions of images, identify elements within them, and use these elements as part of its query-response mechanism.
Unlike traditional LLMs that focus solely on text, Ferret’s integration of visual elements into its processing sets it apart. It can provide contextual responses by leveraging both the textual and visual information. For instance, when asked about an object within an image, Ferret recognizes the object and uses surrounding elements to provide deeper insights or context.
The open-source nature of Ferret is a significant departure from Apple’s usual approach. By making Ferret available to the broader AI community, Apple invites collaboration and innovation, potentially accelerating the development and application of multimodal AI technologies.
How Does Apple Ferret LLM Work?
Apple’s Ferret LLM is a marvel of modern AI, blending language and image processing in a way that’s groundbreaking. At its core, Ferret is designed to understand and interpret both text and visual data, setting it apart from traditional language models. This multimodal approach allows Ferret to analyze specific regions within images, identifying and referring to elements in any free-form shape.
The real magic of Ferret lies in its ability to establish a grounding for text that is deemed groundable by the model. This means that Ferret can connect textual descriptions to specific parts or aspects of an image, enabling a more nuanced and detailed understanding of both the text and the image. For example, if given a picture of a street scene and asked about a particular object in the image, Ferret can identify and provide information about that object, considering its context within the scene.
Ferret’s capabilities extend beyond mere object recognition. It can draw connections between different elements within an image and formulate responses to user queries that involve both the visual and textual data. This opens up possibilities in fields like image search and accessibility, where understanding the context and details of visual content is crucial.
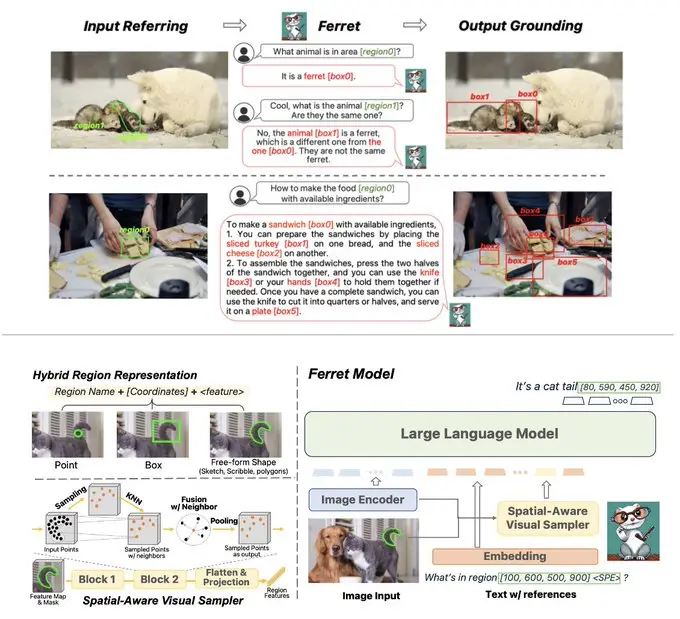
Challenges For Ferret
Despite its innovative features, Ferret faces several challenges that could impact its effectiveness and widespread adoption. One of the primary challenges is scaling the model against larger, more established models. Ferret’s unique capabilities require significant computational resources and sophisticated infrastructure, which could pose hurdles in terms of deployment and scalability.
Another challenge lies in the realm of data and privacy. As with any AI model that processes personal data, there are concerns about how user data is handled, especially given the sensitive nature of visual data. Ensuring privacy and security while maintaining the model’s effectiveness will be a crucial balancing act for Apple.
Additionally, Ferret, like most multimodal LLMs, may produce harmful or counterfactual responses. This necessitates ongoing efforts to refine the model, improve its accuracy, and reduce biases. The open-source nature of Ferret invites collaboration from the AI community, but it also means that Apple will need to manage contributions and updates effectively to maintain the model’s integrity and reliability.
Finally, there’s the challenge of integrating Ferret into Apple’s ecosystem in a way that enhances user experience without compromising performance or privacy. The potential applications of Ferret in Apple devices are vast, but realizing these possibilities will require careful planning and execution.
Apple Ferret LLM’s Potential Influences On iPhones and Other Apple Devices
Enhanced Siri Capabilities
With Ferret’s integration, Siri could undergo a transformative upgrade. Imagine Siri not just responding to voice commands but also understanding and interpreting visual data from your photos or live camera feed. This could lead to more interactive and helpful responses, such as identifying objects in your photos or providing context about a scene you’re capturing. The potential for a more intuitive and visually aware Siri could redefine our interaction with Apple devices, making them more helpful in our daily lives.
Revolutionizing Accessibility Features
Ferret could significantly enhance the accessibility features on Apple devices. For visually impaired users, Ferret’s ability to describe and interpret images could offer a new level of interaction with their devices. It could describe the contents of a photo, read text from images, or even help navigate through complex visual environments. This advancement could make Apple devices more inclusive, providing a richer experience for all users.
Augmented Reality and Gaming
Ferret’s capabilities could be a game-changer for augmented reality (AR) and gaming on Apple devices. By understanding both text and images, Ferret could enable more sophisticated AR applications, where virtual elements interact seamlessly with the real world. In gaming, this could lead to more immersive experiences, with games that adapt to the player’s environment in real-time, offering a unique and personalized gaming experience.
Is Ferret A Commitment to Open and Responsible AI or Strategic Power Play?
The release of Ferret as an open-source project marks a significant shift in Apple’s strategy. This move could be seen as a commitment to open and responsible AI, fostering collaboration and innovation in the AI community. By making Ferret open-source, Apple invites researchers and developers to contribute, potentially leading to more ethical and unbiased AI solutions.
However, it’s also plausible to view this as a strategic maneuver. In a landscape dominated by tech giants like Google and Microsoft, open-sourcing Ferret could be Apple’s way of attracting global talent and accelerating development, thus gaining a competitive edge. This strategy could also be a response to the growing demand for more transparent and ethical AI practices in the industry.
Conclusion
Apple’s Ferret LLM is more than just a new technology; it’s a statement about the future direction of AI development. Whether viewed as a commitment to open AI or a strategic play, Ferret’s impact on the tech world is undeniable. Its potential to transform how we interact with Apple devices, from enhanced Siri capabilities to revolutionary accessibility features, is immense. As we look forward to seeing how Ferret evolves and integrates into our daily tech, one thing is clear: Apple is not just following trends in AI; it’s seeking to redefine them. The journey of Ferret will be a fascinating one to watch, as it may set new standards for AI development and application in the tech industry.