Meta’s V-JEPA Can Achieve YouTube Videos Learning
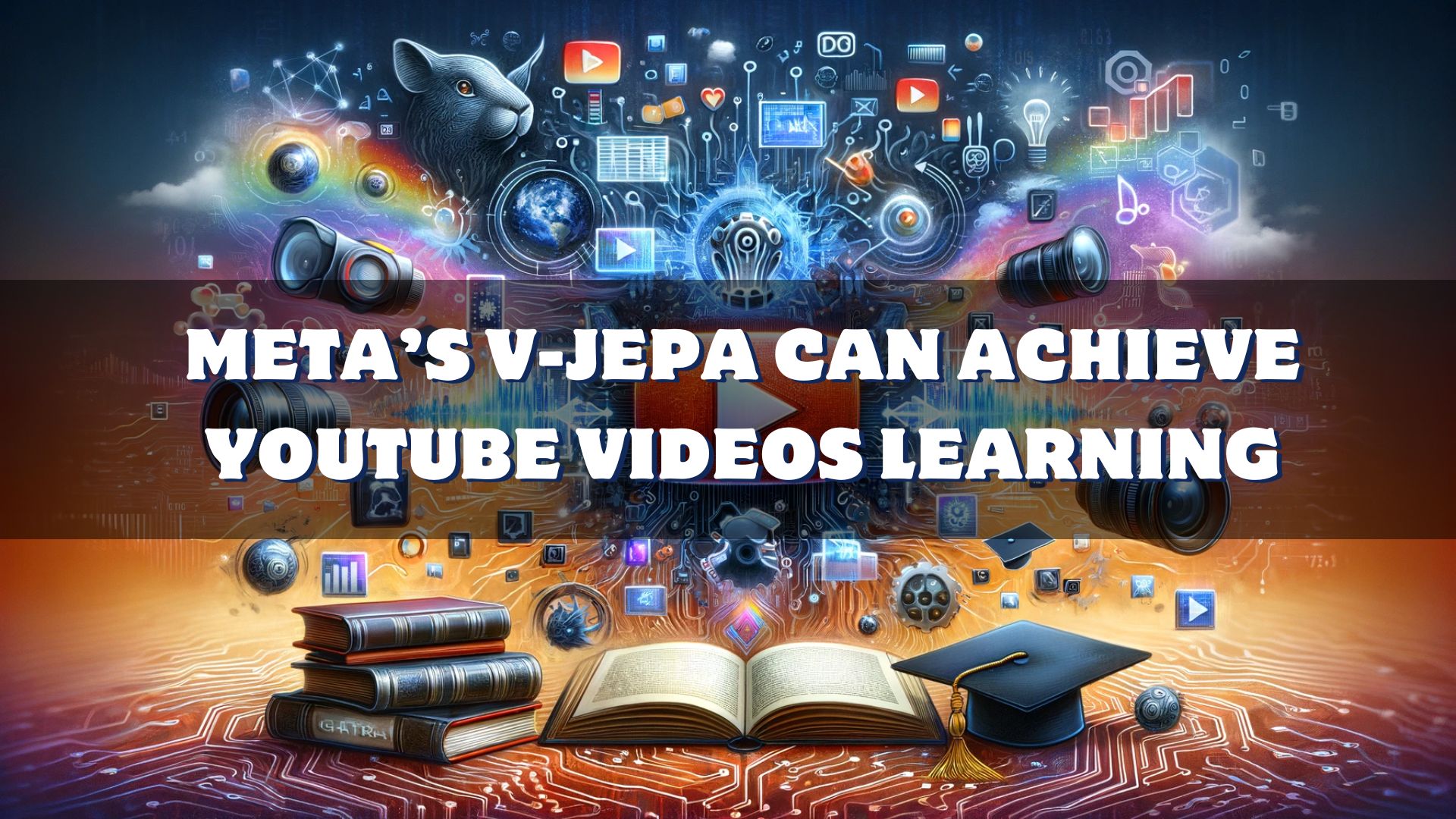
In the digital age, the quest for AI models that can interpret and learn from video content as humans do has been relentless. Meta’s unveiling of the V-JEPA AI model marks a significant milestone in this journey. This introductory section will set the stage for the discussion on how V-JEPA is revolutionizing the way AI understands and learns from video content.
Meta’s V-JEPA, a groundbreaking AI model, is setting new standards in how machines learn from video content. By employing a predictive architecture that focuses on abstract concepts and relationships, V-JEPA offers a more efficient and intuitive approach to video learning. Its ability to process unlabeled data and adapt to various tasks makes it a versatile tool with wide-ranging applications, from enhancing video recommendation systems to advancing research in embodied AI. As we stand on the cusp of new discoveries in video learning AI, V-JEPA paves the way for more intelligent, adaptable, and human-like AI systems.
Table of Contents
What Is V-JEPA?
V-JEPA, or Video Joint Embedding Predictive Architecture, represents a paradigm shift in AI’s approach to video learning. Unlike traditional models that rely heavily on generative methods, V-JEPA employs a non-generative, predictive approach to understand videos. This section will delve into the architecture and philosophy behind V-JEPA, highlighting its departure from conventional models.
How Does V-JEPA Work?
At the heart of V-JEPA’s innovation is its unique approach to processing and understanding video content. Unlike traditional AI models that analyze videos frame by frame, pixel by pixel, V-JEPA adopts a more holistic and abstract method. It operates on the principle of predictive architecture, focusing on the relationships and interactions between objects within a video rather than the individual pixels.
V-JEPA employs a combination of meta-learning and self-supervised learning techniques. Meta-learning, often described as “learning to learn,” enables V-JEPA to apply knowledge gained from one task to new, unseen tasks, thereby improving its adaptability. This is complemented by self-supervised learning, where the model generates its own labels from the video data. This is achieved by masking certain parts of the video and predicting the missing information, much like filling in the blanks in a sentence.
This predictive approach allows V-JEPA to understand the context and dynamics of videos, recognizing patterns and inferring actions without the need for extensive labeled datasets. By focusing on the essence of the content and the interplay of elements within it, V-JEPA can grasp complex scenarios and interactions in a more human-like manner.
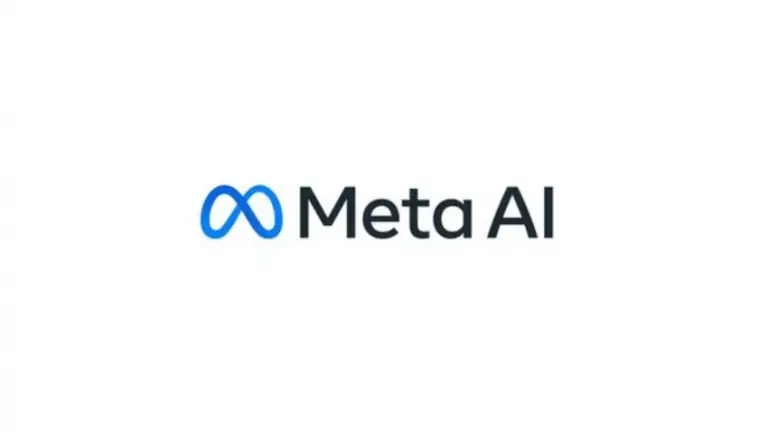
V-JEPA Key Features
V-JEPA stands out due to several key features that distinguish it from conventional video analysis AI models:
- Efficiency in Training: V-JEPA’s ability to learn from unlabeled data significantly reduces the time and resources required for training. By leveraging self-supervised learning, it can understand and predict video content without the need for meticulously labeled datasets.
- Abstract Understanding: Instead of relying on detailed pixel analysis, V-JEPA captures the abstract concepts and relationships within videos. This enables it to comprehend complex interactions and scenarios, facilitating a deeper understanding of video content.
- Adaptability: Through meta-learning, V-JEPA can quickly adapt to new tasks and domains. This flexibility makes it an invaluable tool for a wide range of applications, from content analysis to interactive AI systems.
- Predictive Capability: V-JEPA’s predictive architecture allows it to anticipate actions and outcomes in videos. This capability is crucial for applications requiring foresight and planning, such as autonomous systems and advanced simulation models.
Use Cases of V-JEPA
The innovative features of V-JEPA open up a plethora of applications across various fields:
- Content Creation and Editing: V-JEPA can assist in the automatic generation and editing of video content by understanding and predicting narrative structures and visual elements, streamlining the production process.
- Education and Training: In educational settings, V-JEPA can be used to create interactive learning materials, analyze educational videos for content summarization, and even provide personalized learning experiences based on the student’s engagement and understanding.
- Surveillance and Security: V-JEPA’s ability to interpret complex scenarios and predict outcomes makes it ideal for surveillance systems, where it can identify suspicious activities or anomalies without human supervision.
- Healthcare: In the medical field, V-JEPA can analyze procedural videos to assist in training or to provide real-time guidance during procedures, enhancing both education and patient care.
- Entertainment and Gaming: In the entertainment industry, V-JEPA can be used to create more immersive and interactive experiences, such as in video games where AI characters learn and adapt to the player’s actions.
- Research and Development: V-JEPA’s adaptability and efficiency make it a valuable tool for researchers, enabling the analysis of complex video datasets across various scientific domains, from environmental studies to behavioral science.
By harnessing the power of V-JEPA, industries and researchers can unlock new levels of efficiency, creativity, and insight, pushing the boundaries of what’s possible with AI in video analysis and beyond.
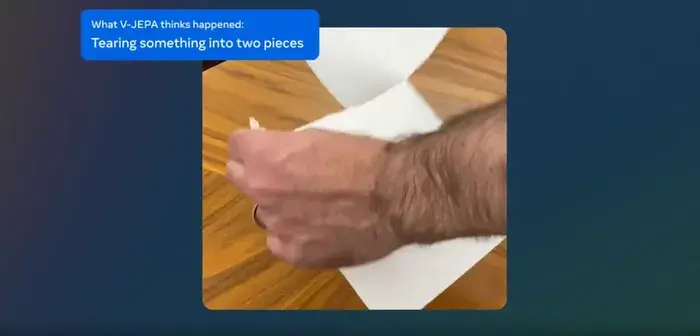
Is Training V-JEPA Difficult?
Training V-JEPA is remarkably streamlined compared to traditional video analysis models, thanks to its innovative learning mechanisms. The model’s reliance on self-supervised learning significantly reduces the need for extensive, labeled datasets, which are often a major bottleneck in AI training. Instead, V-JEPA learns by predicting the missing parts of the video content, a process that mimics human learning and allows for a more natural and efficient training progression. Furthermore, its meta-learning capability enables V-JEPA to quickly adapt to new tasks using knowledge gained from previous ones, enhancing its learning efficiency. Therefore, while V-JEPA embodies sophisticated AI technology, its training process is designed to be as accessible and efficient as possible, making it less daunting for AI practitioners and researchers.
Future of Video Learning AI
The advent of models like V-JEPA heralds a transformative era for video learning AI, promising advancements that could redefine our interaction with digital content. In the future, we can anticipate AI systems that not only understand video content with human-like precision but also generate and manipulate video in creative and interactive ways. The integration of multimodal learning, combining video with audio and textual analysis, will lead to more comprehensive and nuanced AI understanding. Additionally, real-time video analysis capabilities will enhance applications in surveillance, autonomous vehicles, and real-time decision-making systems. As AI models become more adept at learning from and interacting with video content, we will see a convergence of virtual and physical experiences, opening up new dimensions in entertainment, education, and communication.
Bottom Line
V-JEPA represents a significant leap forward in the field of video learning AI, offering a glimpse into the future where AI can learn from and interact with video content in complex, human-like ways. Its innovative approach to learning, combined with its efficiency and adaptability, positions V-JEPA as a pivotal model in advancing AI’s capabilities. As we continue to explore and expand the boundaries of what AI can achieve with video learning, V-JEPA stands as a testament to the potential for AI to not only understand our world but also enhance our interactions within it.
Read More: Apple Vision Pro’s Deepfake AI App
FAQ
Currently, V-JEPA focuses on visual content, but future iterations may incorporate audio for a more holistic understanding.
V-JEPA has been made accessible to researchers and developers, with its code available on platforms like GitHub.
While primarily designed for pre-recorded video content, ongoing advancements may extend V-JEPA’s capabilities to real-time video analysis.