Meta AI's Shepherd: A Deep Dive into Critiquing LLM Generations
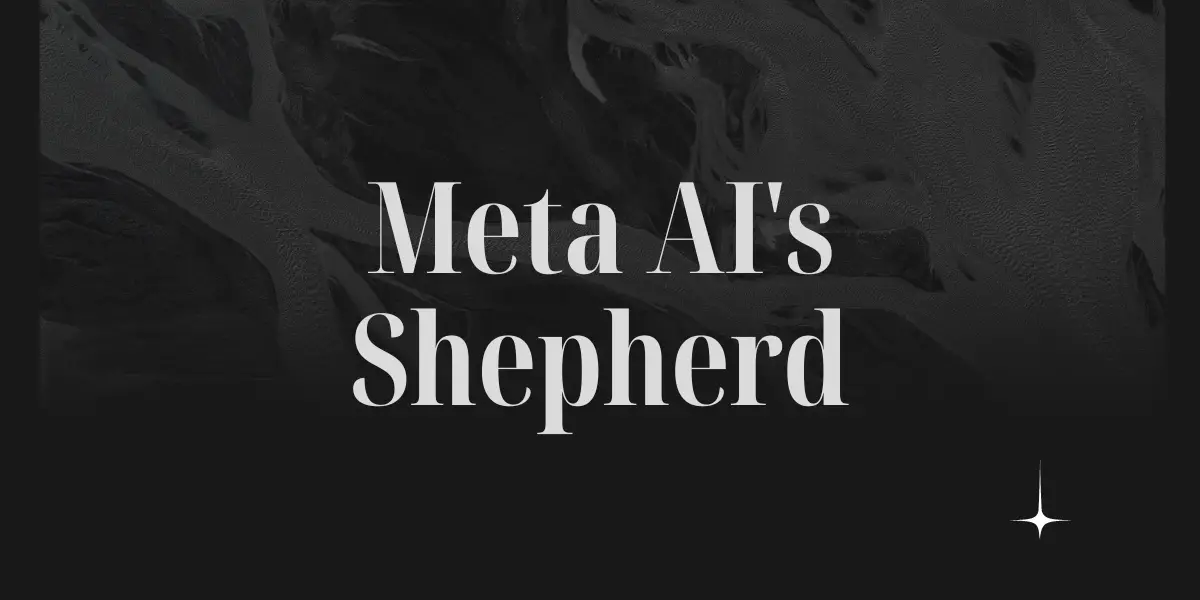
In the realm of artificial intelligence, Large Language Models (LLMs) have become a topic of intense discussion. Capable of generating coherent, contextually relevant, and semantically meaningful text, these models, however, often produce outputs that are inaccurate or questionable. Addressing this challenge, researchers from Meta AI introduce Shepherd, a novel AI model designed specifically to critique and evaluate the outputs of other models.
Table of Contents
The Challenges of Large Language Models
With technological advancements, LLMs have made significant strides in text generation. Yet, these models frequently yield results that are inaccurate, dubious, or nonsensical. This inconsistency underscores the importance of continuous evaluation and improvement of their outputs.
See more:How about Invest in Meta Stock?
From Generic Feedback to Specific Critiques
While traditional research methodologies provided generic feedback on model outputs, they often fell short when dealing with complex tasks like mathematics and reasoning. In contrast, Shepherd offers a more nuanced and in-depth self-feedback mechanism for the responses generated by LLMs.
The Essence of Shepherd
Designed by researchers at Meta AI, Shepherd stands out as a language model optimized to assess and critique outputs from other models. It aims to pinpoint specific issues in the outputs, such as factuality, logical flaws, coherence, and alignment, offering suggestions for modifications when necessary.
Training and Data Behind Shepherd
For training and evaluation, Shepherd leverages two distinct datasets: community feedback and human-annotated input. Community feedback, sourced from online forums, captures a broader spectrum of interactions. In contrast, human-annotated input spans multiple tasks, providing a rich training ground for the model.
Comparing Shepherd with Other Models
Shepherd was benchmarked against other leading models like Alpaca, SelFee, and ChatGPT. The results indicated that feedback from Shepherd was often more accurate and insightful. For instance, while Alpaca frequently praised every model answer, it overlooked potential issues.
What Sets Shepherd Apart
Shepherd’s uniqueness lies in its ability to offer profound feedback across a variety of tasks. Moreover, its performance is further enhanced when fine-tuned with high-quality human-annotated data.
Practical Applications and Impact of Shepherd
Beyond critiquing, Shepherd effectively elevates the quality of content generated by LLMs. This advancement is pivotal for enhancing the reliability and accuracy of AI technologies.
Contributions to Future AI Research
Beyond serving as a valuable tool for the current AI community, Shepherd’s research has also birthed a high-quality feedback dataset. This contribution promises to catalyze further advancements in the field.
Also read:Nvidia Workbench:Training AI Within Reach of Anyone
Conclusion
The introduction of the Shepherd model paves a new path for evaluating and critiquing Large Language Model outputs. Its analytical and critiquing prowess ensures the accuracy and reliability of AI technologies in text generation. As technology continues to evolve, we anticipate witnessing more such innovations that add immense value to our lives and work.