AI Chatbots Can Infer Personal Details from the Text You Type
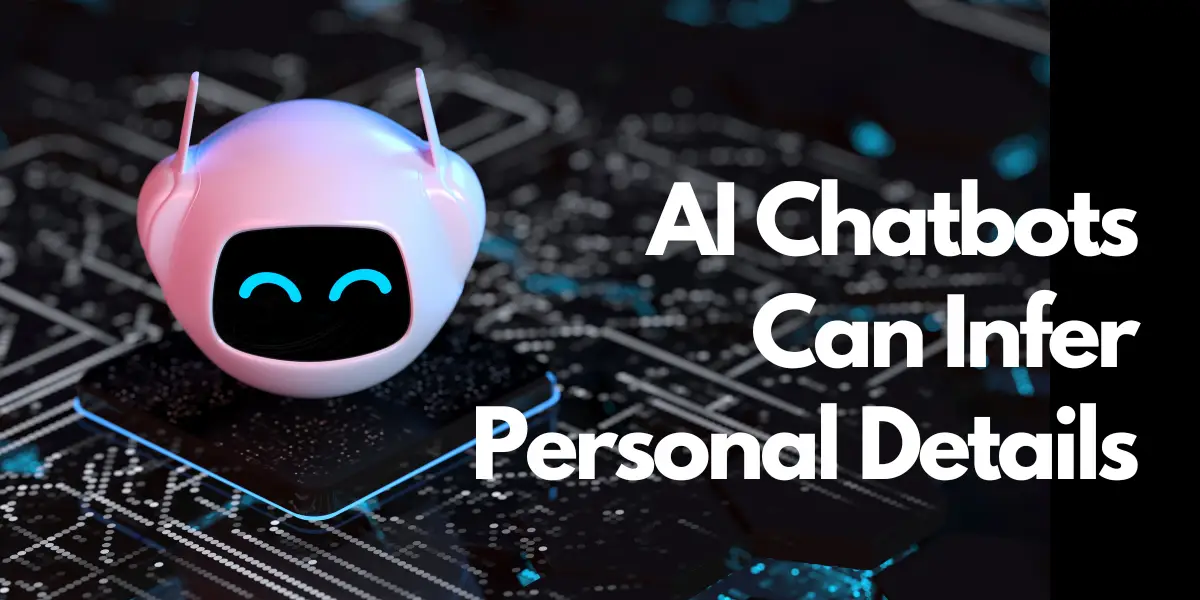
Based on recent research, popular Large Language Models (LLMs) can accurately guess a user’s race, occupation, or location by analyzing seemingly trivial chat content. This phenomenon has sparked a new round of discussions on privacy protection and information security.
This article takes an in-depth look at how large-scale language models (LLMs) such as ChatGPT can reveal intimate information such as an individual’s geographical location, health status, and financial status by analyzing the content of seemingly ordinary conversations. The article highlights the potential risks of this technology and discusses necessary countermeasures to protect privacy and prevent misuse of information.
Table of Contents
The Information Inference Capabilities of LLMs
LLMs, equipped with advanced algorithms, are capable of parsing through vast amounts of data, identifying patterns and information that are not immediately obvious. For example, when researchers recently input the same line of text into OpenAI’s GPT-4, the model was able to accurately infer that the user lived in Melbourne, Australia. The clue was the author’s use of the term “hook turn.” In another case study, a user’s comments about weather preferences allowed the LLM to accurately deduce and pinpoint their exact location, down to the city level. This was possible because the model could cross-reference the mentioned weather patterns with geographical data it had been trained on.
Moreover, LLMs can make startlingly accurate inferences about a person’s health status or financial situation based on seemingly innocuous comments. In one instance, a user’s casual mention of specific shopping habits and budgeting led the LLM to correctly infer the user’s economic bracket.
Risks of Privacy Leaks
The risks extend beyond simple data inference. In situations where users believe their communications are private or anonymous, LLMs can piece together user identities. For example, whistleblowers participating in confidential company surveys could be identified based on their writing style or specific anecdotes they share, even if they don’t provide any overtly identifying information.
In another case, individuals participating in online support groups, believing in the anonymity provided, shared personal experiences. An LLM, analyzing the text, could identify users based on the unique experiences or the manner of speech, potentially exposing sensitive health information or personal struggles.
Potential for Abuse
The potential for abuse is vast, particularly with entities that might use these inferences for targeted advertising, political campaigning, or even more nefarious purposes like stalking or identity theft. For instance, there have been cases where insurance companies use data inferred by LLMs to identify potential customers or even to adjust policy terms based on information that an individual might not have willingly shared.
Another alarming scenario is in authoritarian regimes using LLMs to monitor citizens’ communications, identifying dissenters not just through the content of their messages, but through the style and patterns in their communication, leading to suppression of free speech and even persecution.
Countermeasures and Future Threats
The researchers emphasize that beyond the current privacy concerns, a greater threat may be looming. Soon, internet users may regularly interact with multiple personalized LLM chatbots. Skilled bad actors could potentially “steer conversations” to subtly coax users into relinquishing more personal information to those chatbots without even realizing it.
To delve deeper into this issue, we need to consider the following aspects:
1. Ethical Boundaries of Data Collection
During the training of LLMs, clear ethical guidelines must be established to ensure the protection of individual privacy. This includes restrictions on the types and sources of data that can be used to train models.
2. Strengthening Legal Frameworks
There needs to be a robust legal framework regulating the use of these technologies, especially when handling sensitive information. This could include severe penalties for individuals or entities that abuse LLM technology.
3. User Education and Awareness
Users need to understand the risks they may face when interacting with these advanced systems. This means extensive education and awareness campaigns are necessary to help people understand the importance of protecting their privacy.
4. Technological Countermeasures
Research and development teams should explore technical solutions to reduce the risk of privacy breaches. This may include improving the way models are trained to lessen dependence on sensitive information.
Conclusion
While LLM technology brings immense potential, it also raises concerns about privacy violations and potential abuse. By establishing ethical guidelines, strengthening legal frameworks, raising user awareness, and exploring technological countermeasures, we can balance the relationship between innovation and personal privacy protection. In this evolving field, ongoing dialogue and prudent consideration are crucial.